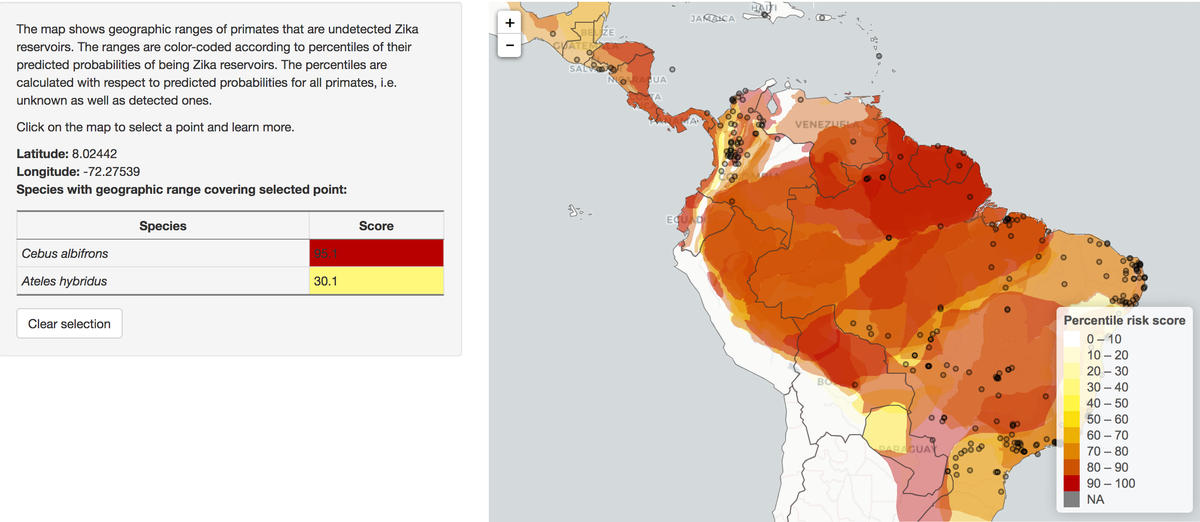
Imagine if we could forecast disease risk like weather.
Zoonotic diseases like Ebola and Nipah virus often take us by surprise. Spillover events occur, and the public health community is left scrambling to contain the disease and minimize suffering. Cary researchers are paring Big Data and machine learning to develop tools that forecast outbreaks before they strike.
They have proposed an early warning system that would shift the infectious disease paradigm from reactive – where first responders scramble to contain outbreaks – to preemptive management of risk.
The challenge
- Zoonotic’ diseases originate in animals. People get sick when we come in contact with body fluids of infected animals or are bitten by an infected vector, such as a tick or mosquito.
- Climate change is shifting the range of animals, vectors, and pathogens.
- Development, agriculture, and population growth are putting people in close contact with animals.
- Trade, travel, and migration can move pathogens into areas with suitable animal hosts, sparking a spillover event.
We are exploring...
- How global data sets on environmental conditions, animal ecology, and zoonotic disease can be mined using artificial intelligence, a form of machine learning, to map areas at risk of spillover events.
- The new models needed to read data that has gaps, so that we can forecast disease globally, not just in regions with deep datasets on animal ecology.
Our discoveries
While many potential bat species likely to transmit Ebola and other filoviruses are found in sub-Saharan Africa, they also range across Southeast Asia and Central and South America.
“Using machine learning methods developed for artificial intelligence, we brought together data from ecology, biogeography, and public health to identify bat species with a high probability of harboring Ebola and other filoviruses globally. Findings highlight new potential hosts and geographic hotspots worthy of surveillance.” – Dr. Barbara Han
In the Americas, primate species likely to harbor Zika – and potentially transmit the virus – are common, abundant, and often live near people. In collaboration with IBM Research’s Science for Social Good initiative, we identified new primates species that are likely to carry Zika virus. This was done by melding two modeling techniques to overcome limited data on primate biology and ecology. Results include an interactive risk map.
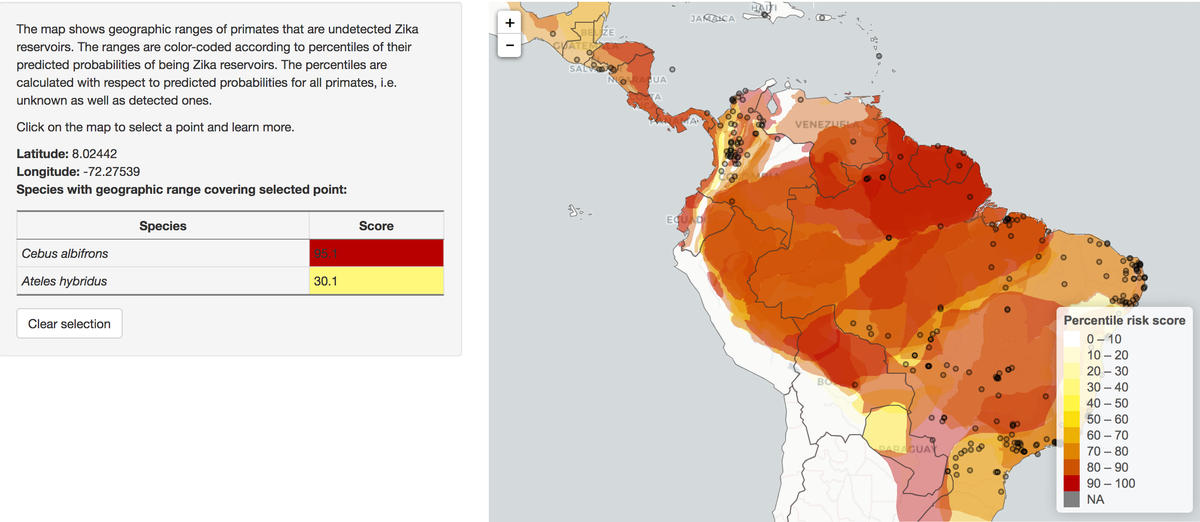
Cary-led machine learning models can pinpoint rodent species that harbor diseases and geographic hotspots vulnerable to new parasites and pathogens. Results equip us with a watch list of high-risk rodent species. The majority of new reservoir and hyper-reservoir species are predicted to occur the temperate zones.